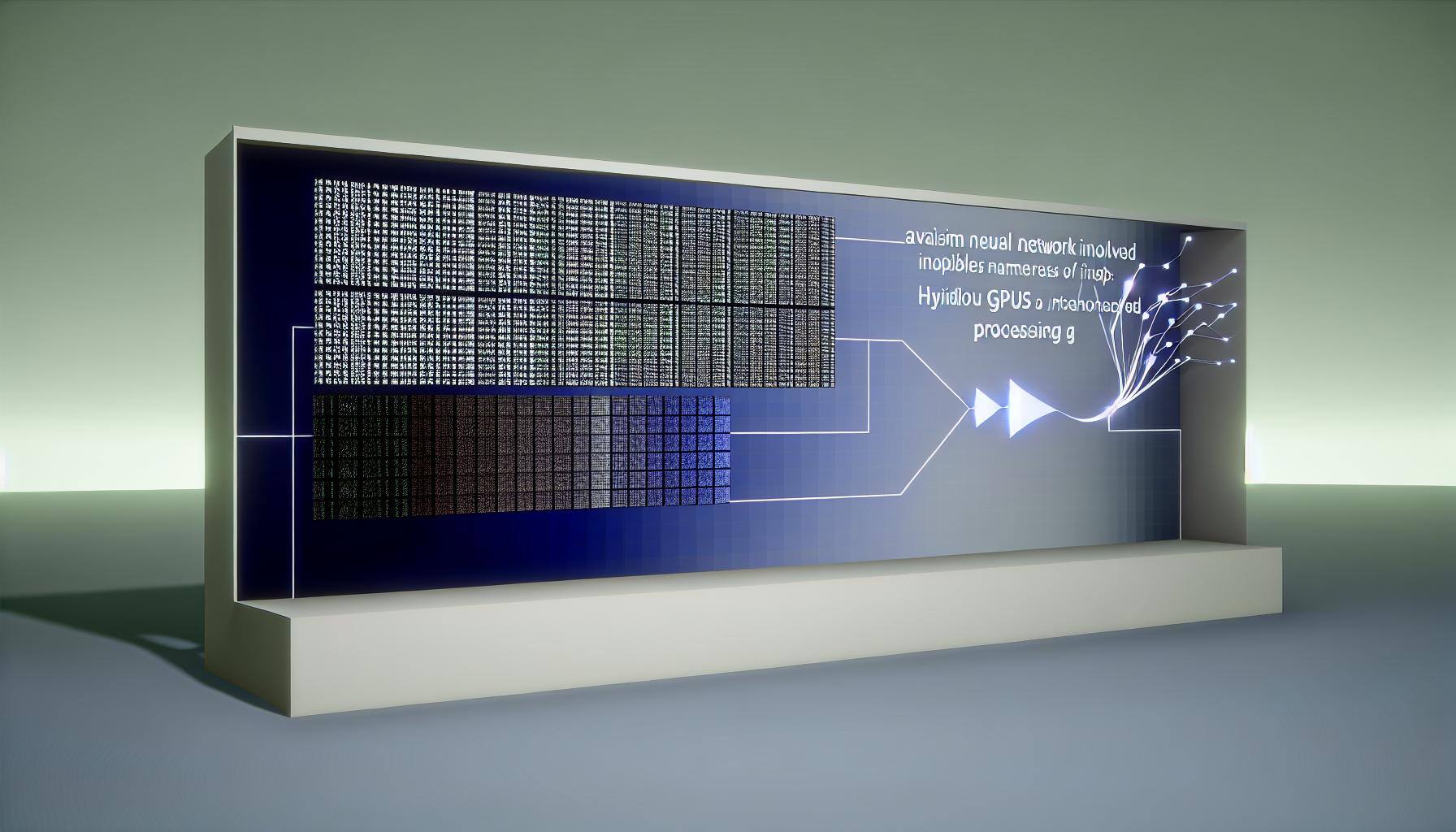
Artificial Intelligence (AI) is rapidly evolving, and at the core of this evolution are foundation models. These are large-scale machine learning models that serve as the bedrock for a wide range of AI applications. In this blog post, we’ll dive deep into what foundation models are, how they are trained, and their diverse applications across industries. We’ll also explore their significance and potential future directions.
What Are Foundation Models?
Foundation models are pre-trained AI models that can be adapted or fine-tuned for specific tasks. Unlike traditional AI models, which are trained from scratch for a particular application, foundation models are trained on vast amounts of data to perform a wide variety of tasks. This makes them incredibly versatile and powerful.
A well-known example of a foundation model is OpenAI’s GPT (Generative Pre-trained Transformer), which is designed to understand and generate human-like text. These models are built on deep learning techniques and have been trained on large datasets that include books, articles, websites, and more. The result is a model that can perform numerous tasks, from answering questions and translating languages to generating creative content like poetry and code.
The Training Process of Foundation Models
Training foundation models is a complex and resource-intensive process. It involves several key steps:
- Data Collection: The first step in training a foundation model is gathering data. For these models, the amount of data required is enormous. They are trained on diverse datasets, including text, images, audio, and video. The data must be comprehensive enough to cover a wide range of topics, contexts, and nuances.For instance, GPT models are trained on datasets that include everything from Wikipedia articles to archived books and forums. This diversity allows the model to understand various forms of language and respond accurately in different contexts.
- Preprocessing the Data: Once the data is collected, it needs to be cleaned and preprocessed. This involves removing any irrelevant information, correcting errors, and ensuring the data is in a consistent format. Preprocessing is crucial because the quality of the data directly impacts the performance of the model.In the case of text data, preprocessing might include tasks like tokenization (breaking text into smaller units like words or sentences), removing stop words (common words like “and” or “the”), and normalizing text (e.g., converting all text to lowercase).
- Training the Model: The training process involves feeding the preprocessed data into a neural network—a type of machine learning model that mimics the human brain’s structure. During training, the model learns to recognize patterns in the data and adjust its parameters accordingly.Training a foundation model requires vast computational resources. It typically involves running the data through the neural network multiple times (a process called epochs) until the model’s predictions become accurate. This process can take weeks or even months and requires powerful hardware, such as thousands of GPUs (Graphics Processing Units).
For example, OpenAI’s GPT-3, one of the largest foundation models to date, was trained on a supercomputer with thousands of GPUs over several weeks. The result was a model with 175 billion parameters, making it capable of performing a wide range of tasks.
- Fine-Tuning: After the initial training, foundation models can be fine-tuned for specific tasks. Fine-tuning involves further training the model on a smaller, task-specific dataset. This step allows the model to specialize in certain areas while retaining its broad, general knowledge.Fine-tuning is often used to adapt foundation models for commercial applications. For instance, a company might fine-tune a model to improve customer service chatbots or develop a specialized tool for analyzing financial data.
Applications of Foundation Models
The versatility of foundation models opens up a wide array of applications across different industries. Here are some notable examples:
- Natural Language Processing (NLP): Foundation models are revolutionizing natural language processing tasks. These include text generation, translation, sentiment analysis, and summarization. Companies use these models to enhance customer service, automate content creation, and improve search engines.For example, chatbots powered by foundation models can provide more accurate and context-aware responses, improving customer interactions. Tools like Grammarly, which offers writing suggestions, also leverage these models to enhance user experience.
- Healthcare: In healthcare, foundation models are being used to analyze medical data, assist in diagnosis, and even predict patient outcomes. These models can process and interpret vast amounts of medical literature, helping doctors stay updated with the latest research and treatments.A notable application is in radiology, where foundation models assist in analyzing medical images. They can detect anomalies, such as tumors, with high accuracy, supporting doctors in making faster and more accurate diagnoses.
- Finance: The financial industry benefits greatly from foundation models, particularly in areas like fraud detection, risk management, and algorithmic trading. These models can analyze large datasets to identify patterns and predict market trends, helping financial institutions make informed decisions.For instance, foundation models can be fine-tuned to analyze financial reports and news articles, providing insights into market movements and helping investors make better decisions.
- Content Creation: Content creation is another area where foundation models excel. From generating articles and marketing copy to creating music and art, these models can assist in producing high-quality content at scale.AI-generated content is increasingly being used by companies to maintain a consistent online presence. For example, news organizations are using foundation models to draft articles on topics like sports and finance, allowing human journalists to focus on more complex stories.
- Education: Foundation models are also making their mark in education by providing personalized learning experiences. They can analyze a student’s learning style and progress, offering tailored recommendations and resources.Educational platforms like Duolingo use AI models to personalize language learning, adapting lessons to the user’s pace and proficiency. This personalized approach helps learners stay engaged and achieve better outcomes.
The Impact and Future of Foundation Models
The impact of foundation models on AI and society is profound. By enabling a broad range of applications, these models are driving innovation across industries and changing how we interact with technology.
However, the rise of foundation models also brings challenges. The computational resources required to train these models are immense, raising concerns about the environmental impact of AI. According to a study by Stanford University, training a large AI model can generate as much carbon dioxide as five cars over their lifetimes. This has led to growing interest in developing more efficient models that require less computational power.
Moreover, there are ethical considerations, particularly around bias and fairness. Since foundation models are trained on vast amounts of data from the internet, they can inadvertently learn and reproduce biases present in the data. This can lead to issues in applications like hiring algorithms, where biased decisions can have significant consequences.
Looking forward, the development of foundation models is likely to continue at a rapid pace. Researchers are exploring ways to make these models more efficient and reduce their environmental impact. There is also ongoing work to address the ethical challenges by improving the transparency and fairness of AI systems.
Another exciting area of research is the development of foundation models that can learn from fewer examples, a concept known as “few-shot learning.” This would allow models to be trained on smaller, more curated datasets, reducing the computational resources required and potentially mitigating some of the ethical concerns.
Conclusion
Foundation models represent a significant advancement in the field of AI, offering the ability to perform a wide range of tasks with high accuracy. Their applications are diverse, spanning industries from healthcare and finance to content creation and education. As these models continue to evolve, they will likely play an even more prominent role in shaping the future of technology.
However, with great power comes great responsibility. As we harness the potential of foundation models, it is crucial to address the challenges they bring, from environmental impact to ethical concerns. By doing so, we can ensure that these models are used to create a better, more equitable future for all.
The journey of foundation models is just beginning, and as we explore their full potential, the possibilities for innovation and positive impact are endless. Whether you’re a developer, a business leader, or simply an AI enthusiast, understanding foundation models is key to staying ahead in the rapidly evolving world of artificial intelligence.
Sources:
- OpenAI’s Research Papers on GPT Models
- Stanford University’s Study on AI Model Training Impact
- Harvard Business Review on AI in Healthcare
- McKinsey & Company Reports on AI in Finance
- IDC’s Analysis of AI Trends and Future Directions