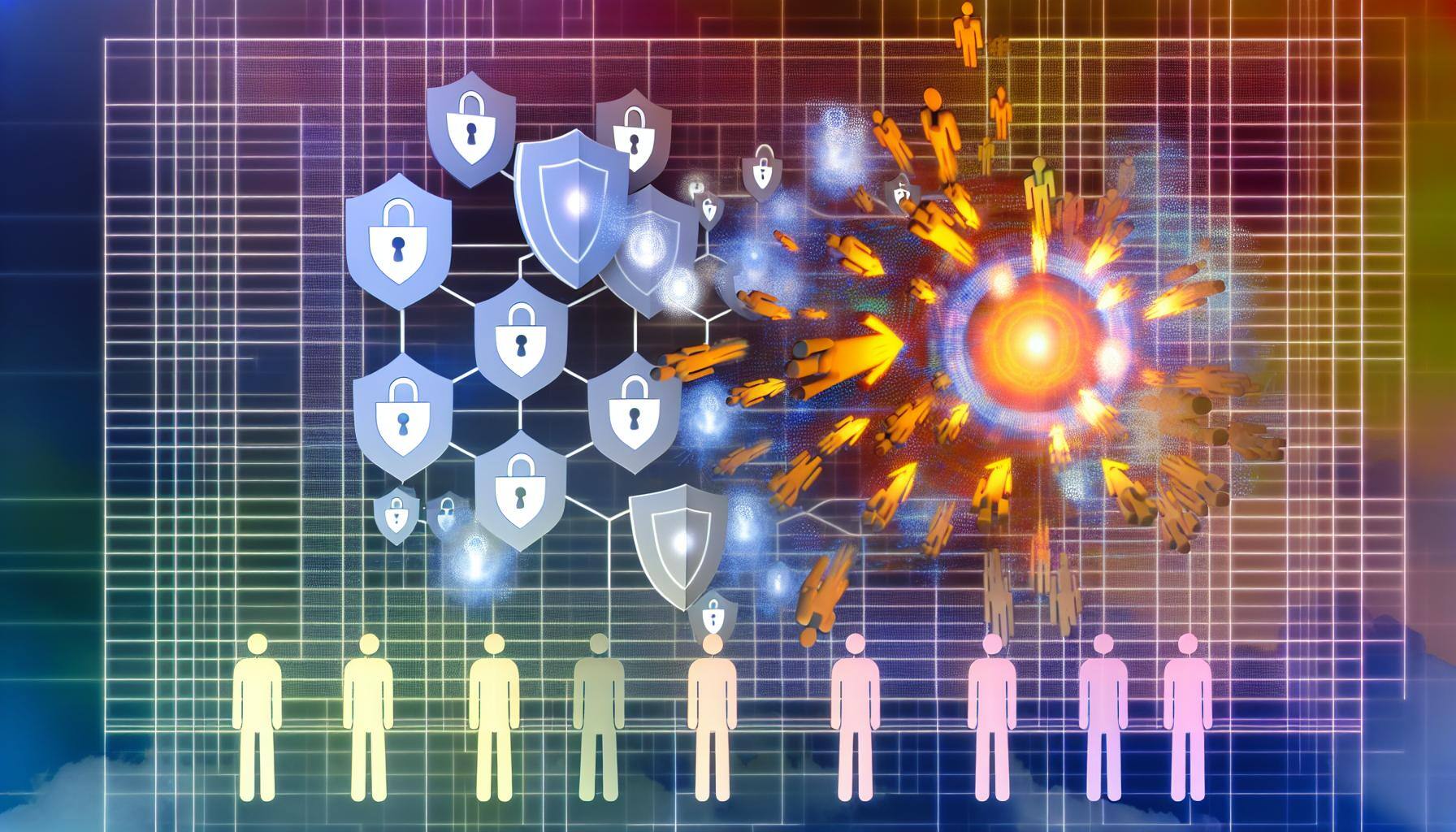
As artificial intelligence (AI) becomes increasingly integrated into various sectors, understanding and mitigating the associated risks is paramount. The MIT AI Risk Repository provides a comprehensive categorization and analysis of AI-related risks, helping stakeholders across industries navigate this complex landscape.
Categorizing AI Risks: A Dual-Taxonomy Approach
The MIT AI Risk Repository organizes risks into two primary taxonomies: Causal Taxonomy and Domain Taxonomy.
- Causal Taxonomy:
- Entity: Identifies the source of the risk, whether it originates from human actors, AI systems, or a combination.
- Intent: Differentiates between risks stemming from intentional misuse of AI and those arising from unintentional consequences.
- Timing: Assesses the stage at which the risk might manifest, be it during development, deployment, or post-deployment.
- Domain Taxonomy:
- Domain: Classifies risks according to the sector or application area, such as healthcare, finance, or governance.
- Sub-domain: Provides a finer categorization within each domain, allowing for a more detailed analysis of risks specific to certain use cases.
Key Insights from the AI Risk Database
The repository includes an extensive database of risks, each categorized by its potential impact and area of occurrence. Some examples include:
- Diffusion of Responsibility: In scenarios where AI systems operate autonomously, the diffusion of responsibility among human operators can lead to severe societal consequences. This risk is classified under human-driven, unintentional actions that occur during the deployment stage, primarily within governance frameworks.
- Algorithmic Bias: Bias embedded in AI algorithms can perpetuate inequality and unfair practices, especially in domains like law enforcement and healthcare. These risks are often unintentional but can cause significant harm if not addressed early in the development phase.
- Governance Failures: Ineffective regulation or oversight in AI applications, particularly in high-stakes areas like autonomous vehicles or financial systems, can lead to catastrophic outcomes. This sub-domain emphasizes the need for robust governance structures.
Utilizing the Repository for Risk Mitigation
Organizations can leverage the MIT AI Risk Repository to proactively identify and mitigate potential risks in their AI initiatives. By understanding the causal and domain-specific factors, businesses can develop tailored strategies that address the most relevant risks in their specific context.
Moreover, the repository’s categorization helps in prioritizing risks based on their potential impact and likelihood, ensuring that resources are allocated efficiently to areas of greatest concern.
Conclusion
The MIT AI Risk Repository is a crucial resource for anyone involved in the development, deployment, or regulation of AI systems. By providing a structured approach to risk identification and categorization, it equips stakeholders with the tools necessary to navigate the complex and evolving landscape of AI risks.